Running Advance demos¶
The same Python and C++ demo applications can be used to run multiple inference models and also work with multiple inputs with just simple changes in the config file.
From a repo of input sources, output sources and models one can define advance dataflows which connect them in various configurations. Details on configuration file parameters can be found in Demo Configuration file
Single input multi inference demo¶
Here is an example of a single-input, multi-inference demo which takes a camera input and run multiple networks on each of them.
root@tda4vm-sk:/opt/edge_ai_apps/apps_python# ./app_edgeai.py ../configs/single_input_multi_infer.yaml
Sample output for single input, multi inference demo is as shown below,
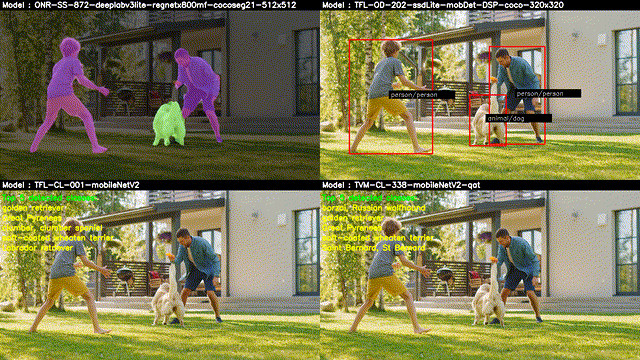
Fig. 12 Sample output showing single input, mutli-inference output¶
We can specify the output window location and sizes as shown in the configuration file,
flows:
flow0:
input: input0
models: [model0, model1, model2, model3]
outputs: [output0, output0, output0, output0]
mosaic:
mosaic0:
width: 800
height: 450
pos_x: 160
pos_y: 90
mosaic1:
width: 800
height: 450
pos_x: 960
pos_y: 90
mosaic2:
width: 800
height: 450
pos_x: 160
pos_y: 540
mosaic3:
width: 800
height: 450
pos_x: 960
pos_y: 540
Multi input multi inference demo¶
Here is an example of a multi-input, multi-inference demo which takes a camera input and video input and runs multiple networks on each of them.
root@tda4vm-sk:/opt/edge_ai_apps/apps_python# ./app_edgeai.py ../configs/multi_input_multi_infer.yaml
Sample output for multi input, multi inference demo is as shown below,
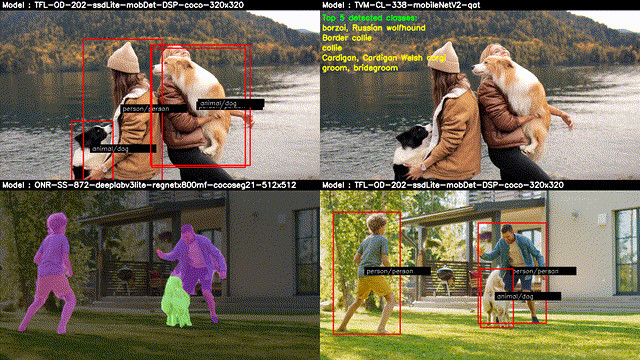
Fig. 13 Sample output showing multi-input, mutli-inference output¶
We can specify the output window location and sizes as shown in the configuration file,
flows:
flow0:
input: input0
models: [model1, model2]
outputs: [output0, output0]
mosaic:
mosaic0:
width: 800
height: 450
pos_x: 160
pos_y: 90
mosaic1:
width: 800
height: 450
pos_x: 960
pos_y: 90
flow1:
input: input1
models: [model0, model3]
outputs: [output0, output0]
mosaic:
mosaic0:
width: 800
height: 450
pos_x: 160
pos_y: 540
mosaic1:
width: 800
height: 450
pos_x: 960
pos_y: 540
We can also share the same model instance to do same inference on differnt inputs. This will reduce the memory foot prints when compred to creating multiple instace of same model. Below is an example for the same,
flows:
flow0:
input: input0
models: [model1, model2]
outputs: [output0, output0]
mosaic:
mosaic0:
width: 800
height: 450
pos_x: 160
pos_y: 90
mosaic1:
width: 800
height: 450
pos_x: 960
pos_y: 90
flow1:
input: input1
models: [model1, model2]
outputs: [output0, output0]
mosaic:
mosaic0:
width: 800
height: 450
pos_x: 160
pos_y: 540
mosaic1:
width: 800
height: 450
pos_x: 960
pos_y: 540
Here model1 and model2 is shared across flow0 and flow1